Unveiling the Power of Nonlinear Dimensionality Reduction in Information Science and Statistics

In the realm of data science, dimensionality reduction techniques play a crucial role in managing high-dimensional datasets and extracting meaningful insights. Linear dimensionality reduction methods, such as Principal Component Analysis (PCA),have been widely used for decades. However, they often fail to capture the complexities and nonlinearities inherent in real-world data.
Nonlinear dimensionality reduction techniques have emerged as a powerful alternative, offering enhanced capability to unravel hidden patterns and relationships in such data. This article delves into the concepts, methods, and applications of nonlinear dimensionality reduction in information science and statistics.
4 out of 5
Language | : | English |
File size | : | 24658 KB |
Print length | : | 326 pages |
Screen Reader | : | Supported |
Mass Market Paperback | : | 138 pages |
Item Weight | : | 5.1 ounces |
Dimensions | : | 5 x 0.32 x 8 inches |
X-Ray for textbooks | : | Enabled |
Conceptual Framework
Dimensionality reduction aims to reduce the dimensionality of a dataset while preserving its essential information. Linear methods project data onto a linear subspace, assuming linear relationships between variables. Nonlinear methods, on the other hand, consider nonlinearities by mapping data into a higher-dimensional space and then projecting it back to a lower-dimensional subspace.
Various nonlinear dimensionality reduction techniques have been developed, including:
- Isomap: Constructs a geodesic distance matrix to represent pairwise distances between data points in a higher-dimensional space.
- Locally Linear Embedding (LLE): Preserves local neighborhood relationships by constructing weights for each data point and its neighbors.
- Laplacian Eigenmaps: Utilizes the eigenvectors of the Laplacian matrix of a weighted graph representing similarities between data points.
- t-SNE (t-Distributed Stochastic Neighbor Embedding): Employs a probabilistic approach to create a low-dimensional representation that preserves local and global relationships.
Applications in Information Science
Nonlinear dimensionality reduction has a wide range of applications in information science, including:
- Data visualization: Reducing the dimensionality of high-dimensional data allows for effective visualization, enabling analysts to identify patterns and outliers.
- Feature selection: By identifying the most informative features, nonlinear dimensionality reduction techniques can aid in feature selection, improving classification and regression models.
- Information retrieval: Nonlinear methods can enhance information retrieval systems by extracting latent topics from text documents and improving document similarity measures.
Applications in Statistics
In statistics, nonlinear dimensionality reduction finds applications in areas such as:
- Clustering: By uncovering nonlinearities, nonlinear methods can improve the accuracy and interpretability of clustering algorithms.
- Manifold learning: Nonlinear techniques can reveal underlying manifolds in high-dimensional data, providing insights into its structure and topology.
- Statistical modeling: Nonlinear dimensionality reduction can be used as a preprocessing step for statistical modeling, improving model performance and interpretability.
Implementation and Evaluation
Implementing nonlinear dimensionality reduction involves selecting an appropriate technique based on the data characteristics and application goals. Several software libraries, such as scikit-learn and manifold, provide implementations of these methods.
Evaluating the performance of nonlinear dimensionality reduction techniques is crucial. Metrics such as distortion, preservation of local and global relationships, and computational efficiency are commonly used for this purpose.
Nonlinear dimensionality reduction has revolutionized the analysis of high-dimensional data in information science and statistics. By capturing nonlinearities and revealing hidden patterns, these techniques empower researchers and analysts to gain deeper insights from complex datasets. As data continues to grow in size and complexity, nonlinear dimensionality reduction will undoubtedly play an increasingly vital role in unlocking the full potential of data-driven decision-making.
References
- Tenenbaum, J. B., de Silva, V., & Langford, J. C. (2000). A global geometric framework for nonlinear dimensionality reduction. Science, 290(5500),2319-2323.
- Roweis, S. T., & Saul, L. K. (2000). Nonlinear dimensionality reduction by locally linear embedding. Science, 290(5500),2323-2326.
- Belkin, M., & Niyogi, P. (2003). Laplacian eigenmaps for dimensionality reduction and data representation. Neural Computation, 15(6),1373-1396.
- van der Maaten, L., & Hinton, G. E. (2008). Visualizing data using t-SNE. Journal of Machine Learning Research, 9, 2579-2605.
Image Alt Attributes
4 out of 5
Language | : | English |
File size | : | 24658 KB |
Print length | : | 326 pages |
Screen Reader | : | Supported |
Mass Market Paperback | : | 138 pages |
Item Weight | : | 5.1 ounces |
Dimensions | : | 5 x 0.32 x 8 inches |
X-Ray for textbooks | : | Enabled |
Do you want to contribute by writing guest posts on this blog?
Please contact us and send us a resume of previous articles that you have written.
Book
Novel
Page
Chapter
Story
Genre
Reader
Library
Paperback
Magazine
Newspaper
Paragraph
Sentence
Glossary
Foreword
Synopsis
Annotation
Footnote
Scroll
Narrative
Biography
Encyclopedia
Dictionary
Character
Resolution
Librarian
Catalog
Stacks
Archives
Study
Lending
Reserve
Reading Room
Rare Books
Special Collections
Literacy
Study Group
Thesis
Dissertation
Theory
A C Deas Ii
Stevie Bowen
William Mortimer Moore
Alyson Belle
Donald E Weatherbee
Vijay Seshadri
David Emerald
2008th Edition Kindle Edition
Nicholas Walker
Mac Mcclure
Andrew Brel
Leeza Hernandez
Peter Stamatov
Laurel J Delaney
Sheila Roberts
Michael Steen
John M Dunn
J R Parker
Dan Coates
Indie Hayes
Light bulbAdvertise smarter! Our strategic ad space ensures maximum exposure. Reserve your spot today!
- Oliver FosterFollow ·11.1k
- George OrwellFollow ·10.9k
- Bret MitchellFollow ·19.3k
- Deion SimmonsFollow ·4k
- Dallas TurnerFollow ·13.3k
- W.H. AudenFollow ·12k
- Tyler NelsonFollow ·14.8k
- Jacques BellFollow ·4.9k
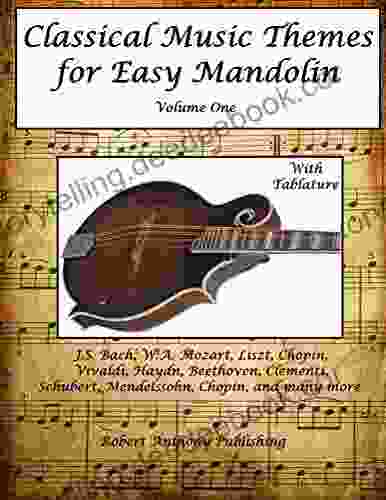

Classical Music Themes for Easy Mandolin, Volume One
Classical Music Themes for Easy Mandolin,...
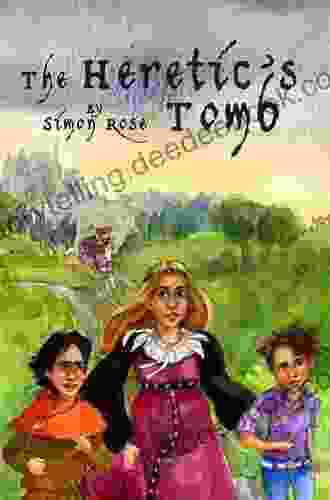

The Heretic Tomb: Unraveling the Mysteries of a Lost...
Synopsis In Simon Rose's captivating debut...
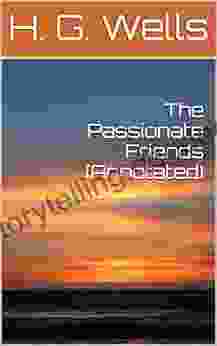

The Passionate Friends Annotated Wells: A Deeper...
Unveiling the...
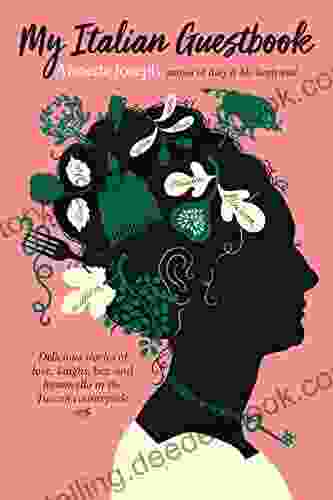

Delicious Stories of Love, Laughs, Lies, and Limoncello...
In the heart of...
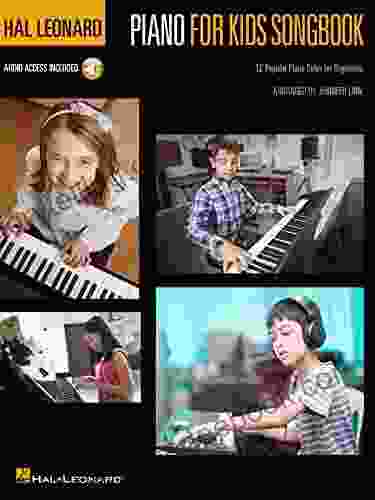

Hal Leonard Piano For Kids Songbook: Unleashing the...
Music holds immense...
4 out of 5
Language | : | English |
File size | : | 24658 KB |
Print length | : | 326 pages |
Screen Reader | : | Supported |
Mass Market Paperback | : | 138 pages |
Item Weight | : | 5.1 ounces |
Dimensions | : | 5 x 0.32 x 8 inches |
X-Ray for textbooks | : | Enabled |